
Understanding how people use their devices often helps in improving the user experience. However, accessing the data that provides such insights — for example, what users type on their keyboards and the websites they visit — can compromise user privacy. We design a system architecture that enables learning at scale by leveraging local differential privacy, combined with existing privacy best practices. We develop efficient and scalable local differentially private algorithms and provide rigorous analyses to demonstrate the tradeoffs among utility, privacy, server computation, and device bandwidth. Understanding the balance among these factors leads us to a Success ful practical deployment using local differential privacy. This deployment scales to hundreds of millions of users across a variety of use cases, such as identifying popular emojis, popular health data types, and media playback preferences in Safari.
Hit the link to read Apple's blog post or click here for the full paper entitled, "Learning with Privacy at Scale".
Share Article:
Facebook, Twitter, LinkedIn, Google Plus, Email, Reddit, Digg, Delicious, StumbleUpon
Follow iClarified:
Facebook, Twitter, LinkedIn, Google Plus, Newsletter, App Store, YouTube
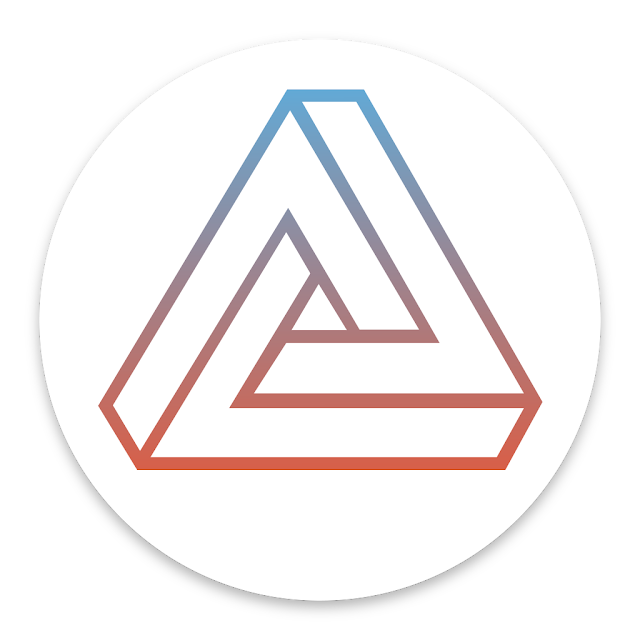
Post a Comment